Understanding and managing sources of variability in cell measurements
Cell Gene Therapy Insights 2016; 2(6), 663-673.
10.18609/cgti.2016.073
Analytical methods are essential for establishing the quality attributes of cell-based products and for monitoring key steps during their production process. They also underpin the development of new cell therapy products (CTPs) by providing insight into biological mechanisms of action and facilitating the research and development that advances manufacturing. Strategies to improve measurement assurance and standards for cell therapy products have increasingly been recognized for their critical roles in streamlining efforts in R&D, translation, and regulatory submissions. Various forums have been created to develop, coordinate, and disseminate industry best practices and experiences in measurement technologies and assay development. Discussions are underway within various standards development organizations to develop standards to ensure quality, improve manufacturing consistency, and provide measurement confidence for cell-based products. In this review, we examine the role of measurement assurance strategies to identify, minimize, and/or monitor sources of variability encountered in measurement processes associated with the discovery and manufacturing of CTPs.
Analytical methods are essential for establishing the chemical, physical, and biological quality attributes of cell-based products, and for monitoring key steps during their production process. Analytical methods also underpin the development of new cell therapy products (CTPs) by providing insight into biological mechanisms of action and facilitating the research and development that advances manufacturing. There is a critical need for analytical methods that can be used to robustly evaluate and compare cell quality attributes from different batches of cells that have, for example, been produced on different days, at different locations, or via a changed manufacturing process. In addition, there remains a need for innovative methods to monitor complex cellular processes as well as methods capable of real time monitoring during the manufacturing process to better understand the impact of process variations and to establish manufacturing controls [1].
The need for improved measurement assurance and standards for CTPs has been recognized as critical for streamlining research, translation, and regulatory submissions. Various forums have been created to develop, coordinate, and disseminate industry best practices and experiences in measurement technologies and assay development. Discussions are underway within various standards development organizations (SDO) to develop documentary standards that improve measurement confidence with an eye to ensure the quality and consistency of component materials, manufacturing processes, and products. Some of these efforts were discussed at the 2014 FDA “Public Workshop: Synergizing Efforts in Standards Development for Cellular Therapies and Regenerative Medicine Products,” which highlighted the need for 1) standards education (i.e., what standards are and how they can help the field), 2) coordination of standards development efforts to achieve maximum return on investment of limited resources and to avoid conflicts and duplications, and 3) data and knowledge to support standards development [2].
As a part of the effort to develop better analytical methods for cell characterization and to generate data that will support standards for advancing CTPs, we recently described [3] a generalized framework for designing and conducting cell measurements that consists of the following elements: 1) clearly defined measurands, i.e., what physical property is being measured that embody quality attributes, 2) well-designed measurements that are qualified and fit-for-purpose, 3) measurements with in-process controls that provides measurement assurance, and 4) appropriate documentation, reporting, and communication. The last element, which is beyond the scope of this paper, concerns the reporting of data values, associated scales (often benchmarked to known value(s)), and uncertainties as well as evidence for confidence and appropriate metadata that collectively enable data comparison. The first two elements of this framework have been previously discussed in the context of cell counting and cell viability measurements [3–5]. Essentially, what has been described is a process for defining the quality attributes of complex cell preparations by breaking it down into component measurements with clearly defined measurands that can be confidently measured. In this report, we examine the role of measurement assurance strategies to identify, minimize, and/or monitor sources of variability encountered in measurement processes associated with the discovery and manufacturing of CTPs. In particular, we discuss elements of the measurement assurance “tool box” and how they may be applied in analytical method design and application, using cell counting as a use case. We conclude with a discussion of bringing shared experiences, best practices, and data into the development of documentary standards and reference materials.
Accuracy & precision for cell measurements
The concepts of accuracy and precision can be described as one’s ability to hit a target with multiple attempts (Figure 1). In this analogy, the ‘true’ value of the biological response resides at the center of the target. Ideally, measurement results are both accurate and precise, that is, they reflect the ‘true’ attributes of the cells; in practice, it may be difficult to obtain high accuracy and precision for many cell measurements because of measurement uncertainties. We consider measurement uncertainties to originate from the entire measurement process and encompass both bias, or systematic measurement errors leading to deviation from the ‘true’ value, and noise, or random measurement errors that cause results to vary in an unpredictable manner. Noise compromises the ability to discern real differences among samples, while bias reduces accuracy. In the worst-case scenario, both accuracy and precision are so poor that the measurement does not provide sufficient discriminatory information for confident decision-making. For cell measurements, noise can be quantified by replicate and repeated measurements; however, accuracy is harder to assess for many cell attributes due to the lack of ground truth.
What make quantitative cell measurements challenging are the complex and highly dynamic nature of cells and cell preparations, the varying sensitivity of cell type and sources to processing steps, and the large number of parameters associated with bioprocessing and measurement processes. Biological variability complicates measurement assurance. The inherent biological differences between different donors may result in different samples or preparations having different ‘true’ values of one or more measurands. Furthermore, different donor samples may have different susceptibilities to processing steps, making the need for in-process controls during the measurement process even more critical.
To approach confidence in interpretation of the results of cell measurements and to enable decision-making, sources that contribute to measurement uncertainty should be understood, minimized, and monitored. Acceptable levels of accuracy and precision will be guided by the biological implications of the measurement result and the practical limitations of the measurement process; this is the essence of ‘fit for purpose.’ Establishment of a robust measurement process with in-process controls will provide added confidence that the result represents the ‘true’ cell attributes.
Measurement assurance toolbox to understand & manage sources of measurement variability for cell measurements
Imaging-based cell counting as a use-case
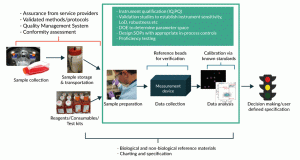
Figure 2. An example of a generalized cell counting process that involves an automated imaging device, and where potential controls and standards for managing and minimizing sources of variability could be used. The central green box contains 3 steps of a typical measurement process; other aspects such as the composition of donor samples, reagent sourcing, and sample handling may contribute additional variability to the cell counting process
Measurement assurance means having sufficient confidence in the measurement results to make decisions with confidence. Measurement assurance can be achieved by a number of strategies. These include, but are not limited to, validated analytical methods, implemented in-process controls, the use of reference materials, and the use of experimental design to systematically examine the quality of a measurement process. It is important to understand the roles of each measurement assurance strategy for the different steps of a measurement process.
Below we examine a generalized automated imaging-based cell counting measurement and how controls and standards can be used to provide more confidence (Figure 2). The measurement process consists of three general steps depicted in the green box: 1) sample preparation, 2) data collection, and 3) data analysis. The sample preparation step may include mixing, diluting, and staining of cells using appropriate reagents, as well as sampling and other common procedures. A commercial “black box” automated image-based cell counting instrument is used to collect the data. The data analysis step may or may not require additional user input for setting parameters such as brightness thresholds, cell size, etc. It is important to consider upstream activities which might impact the measurement process, such as the collection of cells and their subsequent processing, storage and transportation steps, as well as reagents, consumables, and testing kits necessary for this counting measurement process. Each step in the process may introduce sources of variability that can greatly affect the measurement outcome and, as a result, the downstream biological implications and business decisions.
Also indicated in Figure 2 are various measurement assurance tools that can be used to identify and minimize sources of variability. These lists of controls and standards are by no means complete, universally applicable, or required for all cell counting measurement processes. Nevertheless, it is immediately obvious that no single control or standard can assure the quality of the entire cell counting measurement process. Instead, multiple controls and standards are needed to ensure quality at each or several steps of the measurement process. Some controls and standards are intended to be implemented prior to routine measurements, while others are meant to serve as in-process controls or comparators during measurement of the samples.
Formal documentary standards play a variety of roles, such as definition of terms, describing a specific analytical method with or without specific requirements, delineating explicit requirements for certification, defining the requirements of a quality management system, conformity assessment, or proficiency testing. Some documentary standards may invoke the use of a reference material. Note that reference materials are sometimes referred to as standards, such as NIST Standard Reference Materials (SRMs), which are highly qualified materials with certified reference values and uncertainties. Not all reference materials need to be of the highest qualifications. Fit for purpose reference materials can be developed in-house, such as reference cells from an internal master cell bank.
Measurement assurance in the development of analytical methods
The selected analytical method for the measurement of the intended cell attribute should be qualified and validated. Qualification studies should include measurements that establish assay sensitivity, limits of detection (LoD), linearity or proportionality of response, specificity, accuracy and precision, and robustness and sensitivity to experimental parameters. Design of experiment (DOE) can be used to identify the sensitivity of the assay to changes in experimental parameters, such as enzymatic treatment and reagent source. As a systematic approach, DOE can help to establish the acceptable range of assay performance metrics beyond which the assay performance may become questionable. Working within the acceptable performance metrics provides additional confidence of the assay performance.
In the image-based cell counting use case presented here, several preliminary studies may be conducted to select and optimize a procedure that is most appropriate for the intended use. Clear identification of the measurand is critical to optimizing a procedure. A number of measurands may be components of a particular quality attribute. For example, to determine the count of viable cells, the actual measurand may be the detection of dye inside of cells. Alternatively, the measurand may be considered to be ‘the number of cells that have been fluorescently labeled with a dye that is excluded from cells with intact membranes ’ divided by ‘the total numbers of cells fluorescently stained by a dye that stains all cells’. A sensitivity analysis, perhaps using DOE, can reveal the importance of parameters of the measurement system to the measurement result. These parameters may include the limit of detection of the dyes, spatial resolution, spectral specificity, or the ability of algorithms to discriminate between particles of different sizes. Studies of robustness to measurement variables such as inter-operator variability, inter-device variability, inter-day variability, inter-batch variability can be conducted. Ultimately, a set of specified measurement conditions under which a reliable cell counting measurement is expected can be determined and codified in an SOP.
Reference materials
Reference materials can serve to assure various aspects of a measurement process. Reference materials can provide instrument-to-instrument comparability, calibrate a measurement, validate a part of or a complete measurement process, or provide traceability of an analytical value. They may also serve to qualify the correct operation of an instrument and software.
A reference material may be biological or non-biological in nature and may be associated with a reference value determined through rigorous measurement processes and/or orthogonal measurement methods. For example, a bead reference material designed to serve as a calibrant may have a reference value for the bead diameter mode, or a reference values for the concentration range. Specific use of a reference material in cell counting may include beads with a reference concentration for instrument quality control, beads with a reference size distribution to verify microscope focus, or a cell-based reference material to verify multiple steps of a measurement process. A reliable reference material is at minimum validated to be homogeneous and stable over the time of intended use.
Inert reference materials such as polymeric beads are commonly used as surrogates for cells in many cell measurements. Inert reference materials can have advantages over biological reference materials in that they can often be more stable and more homogeneous, better withstand analysis by a range of orthogonal methods, and be more resistant to degradation during the handling and measurement processes. Because of these properties, they provide an excellent immutable reference for instrument response. On the other hand, depending on the measurand, such materials may not embody the necessary biological, optical, and other properties of cells. For example, testing the sensitivity of cellular integrity to various handling steps in an assay protocol will undoubtedly require a cellular reference material. Such shortcomings might necessitate the development of in-house cell based reference materials to assess the performance of a cell counting measurement process. Since cells are less homogeneous and stable than immutable inert materials, additional qualification steps will be needed to assure the reliability of these reference materials.
Biological reference materials can be useful for evaluating the pre-processing steps of a measurement as well as some aspects of the measurement and data analysis steps. Cell based reference materials are challenging to develop and certify because of their complex nature and instability. A few cell-based reference materials do exist, such as the lyophilized CD34+ Cell Enumeration System Suitability Reference Standard from USP and other suppliers [6]. This reference material is based on several years of highly technical measurements [7], and thus illustrates the effort required to produce such reference materials. The fabrication of a stable, homogeneous reference material for every measurement of interest may be impractical, but the development of multipurpose cells may be equally challenging. Even more challenging would be the development of living cells that would constitute a reference material because the consistency of the relevant properties of such a material would likely have to be validated with every use.
A reference material and its associated reference values may only help to qualify or verify a specific aspect of the measurement. Over-interpretation of the results from a reference material may lead to a false sense of confidence for the analytical method. For example, beads with reference count can only assure that the instrument is performing with specification and does not provide sufficient confidence for the instrument’s ability to count cells that may “look” very different from the beads; likewise, reference beads that are intended to verify the appropriate focus in an imaging cell counting instrument cannot verify the appropriate image analysis parameters needed to discriminate cells from debris.
Establishing confidence in cell counting in the absence of a reference material
Generating a universal cell reference material that would embody the characteristics of many different cell types, and be appropriate for assessing measurements from different kinds of instruments, is highly challenging. Different cell types of interest to this field have different optical properties, sizes, shapes, tendencies to generating debris; and a variety of methods are used, which include fluorescence, bright field, and other optical methods, as well as electrical/impedance methods. In response to this challenge, we have recently shown that an experimental design and statistical analysis framework can be used to evaluate the performance of a cell counting measurement process for any method or cell type based on the fundamental concept of proportionality as an internal control [8]. Quantitative metrics are generated and can be used for assay selection, optimization, or comparison. This is not a method that provides an assessment of absolute accuracy, because it does not totally rule out bias that scale with dilution. However, it does allow comparison of methods for relative accuracy and precision, thus providing confidence in the selection of a counting method.
In addition, strategies to assess accuracy may include the use of orthogonal methods and comparing results from different laboratories through inter-laboratory comparison studies. These approaches can help to uncover bias and establishing confidence in the accuracy of the result.
In-process controls for the measurement process
Confidence in a measurement entails insuring that the measurement system is operating as expected. Even when a measurement process has been optimized and validated, the performance of each measurement can be monitored by in-process controls to assure that the measurement has not been compromised by unanticipated changes in measurement components and conditions such as reagents, temperature, operator, etc. In-process controls can be measurements that are conducted at different steps during the measurement process. Charting of monitored results over time provides expectations and specifications for measurement results. Table 1 provides some examples of in-process controls for the automated imaging cell counting use case presented here. In this example, several in-process controls can be derived directly from the image data already collected from the instrument, such as image background, object diameter, extent of aggregation, or spatial distribution of objects. Although these image attributes may not be the final measurand of interest (i.e., total cell count or viable cell count) used in evaluating the biological attribute, they can provide key information on the status of the measurement itself. In some cases, in-process controls may require the use of an additional experiment or control material to monitor a specific aspect of the measurement.
Table 1: Examples of in-process controls for an automated imaging cell counting measurement process. | |||
---|---|---|---|
Aspect to be validated | Specific concerns | In-process control | Specifications |
Reagent quality | Reagents are free of contaminants and precipitants | Running a reagent only control | Object count and object size distribution are within specification for a no-cell control experiment |
Image background intensity and distribution | Imaging chamber is clean and illumination is even across image to ensure proper image analysis | Review of raw image data | Raw image back-ground intensity is within specification |
Object spatial distribution | Flow into the imaging chamber is unob-structed | Review of processed image data | Objects are distributed randomly and within specification |
Object size distribu-tion | No large aggregates or small debris | Review of processed image data | Object sizes are within specification |
Camera focus/ magnification | Image is in focus and magnification is correctly set | Running a control material with known size | A control material of known size is within specification |
A systematic approach to measurement assurance & standards
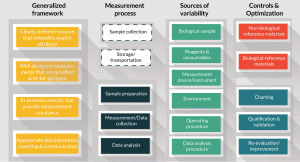
Figure 3. General overview of the measurement process, key steps, sources of variability and controls.
Concepts illustrated in the above cell counting example can be generalized for other cell measurements. A generalized framework is shown in Figure 3, which includes the elements of measurement assurance discussed earlier, as well as aspects of the measurement process, the sources of variability, and controls and standards. Once clearly defined measurands have been established, and fit-for-purpose measurements and assays have been identified, the measurement process can be broken down into three key steps as depicted in the 2nd column of Figure 3: 1) the sample preparation procedure prior to the measurement, 2) data collection, and 3) data analysis. As noted earlier, sample collection, storage, and transportation do not fall under the measurement process per se, but steps to implement these processes are known to influence the cell attribute. Some sources of variability are shown in the third column of Figure 3. Sources of variability are sometimes further dissected and displayed using an Ishikawa or fishbone diagram to evaluate the effects of measurement parameters on measurement results [9]. Identifying the parameters that are the most important sources of variability, and determining the range over which the parameters can vary but the result is robust, can be determined with design of experiment. A good example is the process to minimize variability in measuring the MTS cell metabolic activity assay through design of experiment [9]. Cell attributes are often the result of a complex aggregate of many parameters that may vary in a non-linear fashion. Identifying and controlling all the sources of variability in a biological measurement may be impossible. Design of experiment can help to establish a set of limits within which an analytical method can be robust and will likely return a reliable value for assessing the intended cell attribute.
While reference materials and standards are important in measurement assurance, few have been explicitly developed for cell therapy products thus far. Recognizing this need, efforts are underway to develop international documentary standards and reference materials for CTPs. Within ISO/TC 276: Biotechnology, various topics relevant to bioprocessing are under development, with the aim of assuring the quality and consistency of CTPs; control of ancillary materials, equipment, and transportation, are among the topics being considered for documentary standards. In addition, general considerations for cell measurements, and specific standards for cell counting are under development. Other relevant standards for CTPs exist or are under development within other organizations, industry, and professional consortia [2].
Translational insight
Establishing acceptable levels of accuracy and precision for measurements of cell attributes is necessary for confident decision making. Here, we show a process for thinking about how to identify, minimize, and/or monitor sources of variability by systematically determining where and how to apply measurement assurance strategies. This process is ideally informed by a deep understanding of the biological implications of the measurement result and the limitations of the measurement process. Through a generalized image-based cell counting measurement, we show how controls and standards, including reference materials, in-process controls, the use of experimental design, and qualified and validated SOPs may be used to instill measurement confidence. This general framework is applicable to a broad range of cell measurements and should facilitate the development of consensus standards and reference materials for CTPs.
Acknowledgements
We would like to thank Drs. Michael Halter and Carl Simon for their input.
Financial & competing interests disclosure
The author has no relevant financial involvement with an organization or entity with a financial interest in or financial conflict with the subject matter or materials discussed in the manuscript. This includes employment, consultancies, honoraria, stock options or ownership, expert testimony, grants or patents received or pending, or royalties. No writing assistance was utilized in the production of this manuscript.
References
1. National Cell Manufacturing Consortium. Achieving Large-Scale, Cost-Effective, Reproducible Manufacturing of High-Quality Cells: A Technology Roadmap to 2025, Advanced Manufacturing Technology Consortia (2016).
2. Arcidiacono JA, Bauer SR, Kaplan DS, Sarkar S, Lin-Gibson S. FDA and NIST Collaboration on Standards Development Activities Supporting Innovation and Translation of Regenerative Medicine Products. Cytotherapy (Submitted).
3. Lin-Gibson S, Sarkar S, Ito Y. Defining quality attributes to enable measurement assurance for cell therapy products, Cytotherapy 2016; 18(10), 1241–4. CrossRef
4. NIST Workshop: Strategies to Achieve Measurement Assurance for Cell Therapy Products. May 11-12, 2015. Website
5. Simon CG, Lin-Gibson S, Elliott JT, Sarkar S, Plant AL. Strategies for Achieving Measurement Assurance for Cell Therapy Products. Stem Cells Translational Med. 2016; 5(6), 705–8.
CrossRef
6. USP General Chapter USP Flow Cytometric Enumeration of CD34+ Cells. Website
7. Stebbings R & Wang L. Determination of CD4+ Cell Count per µL in Reconstituted Lyophilized Human PBMC Pre-labeled with Anti-CD4 FITC Antibody. Cytometry 2015; 87A, 244–53 (2015).
CrossRef
8. NIST, Biosystems and Biomaterials Division, Cell Counting Experimental (Created July 7, 2015, Updated August 25, 2016, accessed Nov 8, 2016). https://www.nist.gov/mml/bbd/cell-measurements/advanced-therapies-cellular-and-gene-therapy-and-regenerative-medicine-0-0
9. Rösslein M, Elliott JT, Salit M et al. Use of Cause-and-Effect Analysis to Design a High-Quality Nanocytotoxicology Assay. Chem. Res. Toxicol. 2015; 28(1), 21–30.
CrossRef
Affiliations
Sheng Lin-Gibson*, Sumona Sarkar, John Elliott & Anne Plant
Biosystems and Biomaterials Division, Material Measurement Laboratory, NIST, Gaithersburg MD, USA.
* Author for Correspondence: Sheng Lin-Gibson, slgibson@nist.gov
This work is licensed under a Creative Commons Attribution- NonCommercial – NoDerivatives 4.0 International License.